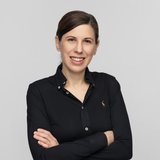
Ap.Prof.in DI Susanne Strohmaier, PhD
Stv. Leitung | Ap.Prof.inTel.: +43 (0)1 40160-34705
E-Mail: susanne.strohmaier@meduniwien.ac.at
Medizinische Universität Wien
Zentrum für Public Health
Abteilung für Epidemiologie
Kinderspitalgasse 15, 1. Stock, Zi. 125a
1090 Wien
Susanne Strohmaier
After graduating with a Dipl.-Ing. degree (equivalent to MSc) in Technical Mathematics from the Karl Franzens University in Innsbruck in 2010 and gaining some initial statistical experience at the Department for Medical Statistics, Informatics and Health Economics, Susanne Strohmaier moved to Lancaster (UK) to continue her studies in Medical Statistics (MSc, 2012).
Between 2012 and 2015 Susanne took her PhD in biostatistics taking part in the Marie Curie Initial Training Network – MEDIASRES, at the University of Oslo (Norway), working on causal mediation analysis techniques.
In 2016 she started her postdoctoral training in epidemiology at the Channing Division of Network Medicine and Harvard Medical School (Boston, USA) where she gained experience in analyzing large scale epidemiological studies. In fall 2018 Susanne returned to Austria to work on her Marie Curie Individual Fellowship project - SCOUT (Supporting Causal Conclusions from Observational Survival Studies) at the Section for Clinical Biometrics (CeMSIIS, now CeDAS, MedUni).
In October 2020 Susanne joined the Department of Epidemiology at the Medical University of Vienna. In 2021 she was admitted into the MedUni internal tenure program which she completed in 2024 as Ap. Prof. Since 2025 she acts as the deputy head of the department.
Education
Dipl.-Ing. (Technical mathematics), Karl Franzens University Innsbruck, 2010
MSc (Statistics), Lancaster University, 2012
Ph.D. (Biostatistics), University of Oslo, 2016
Ap. Prof. (Habilitation equivalent), Medical University of Vienna, 2024
Area(s) of Specialization
- Methods development and application for causal mediation analysis
- Causal inference for time-to-event data
- Open science – quantifying variation due the multiplicity in analysis approaches
- Occupational epidemiology – studying adverse effects of night shift work and sleep behaviors, particularly involving transgenerational studies
Ongoing projects
TOPSTATS-M (Towards precise statistical analysis plans facilitating microdata analyses to advanced Health Research)
VABABS (Improvement and protection of women’s health at a population level by Value Based Breast Screening in Vienna)